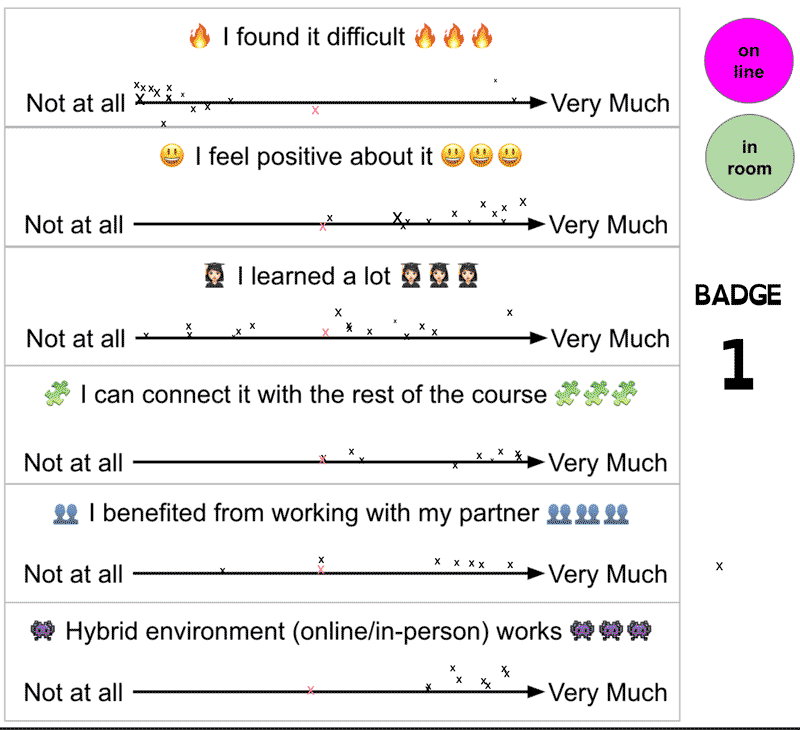
Edinburgh Geoparser Back On The Map
We are delighted to announce the release of the new version (v1.2) of the Edinburgh Geoparser, a tool to geoparser contemporary English text. Most importantly, it now comes with a new map display using OpenStreetMap.
We also made a few fixes to make it run on the latest versions of MacOS and have added instructions of how to visualise the timeline display on different browsers.
The new geoparser also incorporates a gazetteer lookup that’s now supported by the University of Edinburgh Digital Library team. We continue to support queries to all gazetteers that were distributed with the previous release of the geoparser (see the list here).
We are a small research team so updating this technology regularly can be challenging but we hope that with this new release the Edinburgh Geoparser will continue to be useful for place-based research and teaching. More information on how do download the new version and on its updated documentation can be found here.
Reflections on my first PhD Publication at the Second Workshop on Gender Bias in Natural Language Processing
This post originally appeared on Lucy Havens’ blog on 10 February 2021.
This winter (December 2020), I published a new research methodology for Natural Language Processing (NLP) researchers to consider, which I refer to as a bias-aware methodology. Earlier in the year, a couple months into my PhD research on using NLP to detect biases in language, I’d been relieved to see Blodgett et al.’s ‘Critical Survey’ confirm what I’d begun to suspect: NLP bias research was missing the human element. As a researcher new to the NLP domain, I’d been shifting between frustration with the vagueness of existing NLP bias research and doubt in my own understanding. Soon after reading the Survey, I came across Kate Crawford’s 2017 keynote, The Trouble with Bias. Both the Survey and keynote discuss the harmful consequences of siloed technology research, and they both call for interdisciplinary and stakeholder collaboration throughout the development of technology systems. The Survey was published three years after the keynote. Why was there still a need to make the same calls?
I realized that, although there was a wealth of evidence supporting the need for interdisciplinary and stakeholder collaboration, there wasn’t guidance on how to go about engaging in such collaboration. Drawing on my background working at the intersection of multiple disciplines, I went to work creating a new methodology that would outline how to collaborate across disciplines and with system stakeholders. Though my work and studies have fallen under many different names (to name a few: Information Systems, Human-Computer Interaction, Customer Experience, Design Informatics), I consistently situate myself in the same sort of place: at the intersection of groups of people who do not typically work together. I enjoy adapting the tools of one discipline to another to enable new types of research questions to be asked and new insights to be discovered. To adapt one discipline’s tools for another, I listen closely to how people communicate, adopting distinct vocabularies and presentation styles depending on my audience. I employ human-centered design methods, observing and interviewing, even if only informally, to gather information about the goals and concerns of my collaborators. As those involved in anything participatory, user-centered, or customer experience-related have likely experienced, once you’re exposed to the methods, it’s difficult to stop yourself from seeing everything through a human-centered design lens. So, my PhD was inevitably including some form of human-centered design.
In the new methodology I propose with my co-authors in Situated Data, Situated Systems: A Methodology to Engage with Power Relations in Natural Language Processing Research, I’ve embedded interdisciplinary concepts and practices into three activities for researchers to execute in parallel: (1) Examining Power Relations, (2) Explaining the Bias of Focus, and (3) Applying NLP Methods. The practice of participatory action research, which plays a part in all three activities, embeds stakeholder collaboration into the methodology as well. I’m in the process of executing these three activities during my PhD research, so I will certainly refine the methodology over time (I’d also love feedback on how it suits your work and how you’d adjust it!). That being said, the methodology does provide a starting point for all types of NLP research and development, facilitating critical reflection on power relations and their resulting biases that impact all NLP datasets and systems. If your dataset or system has a huge community of potential stakeholders, the methodology asks you to make decisions based on the people at the margins of that stakeholder community, assembling as diverse a group of people as possible with whom you can collaborate. If your project timeline does not allow adequate time for stakeholder collaboration, the methodology asks you to be detailed in the documentation of your work, stating the time, place and people that make up your project context, and the power relations between people in your project context.
NLP uses human language as a data source, meaning NLP datasets are inherently biased, and NLP systems built on those datasets are inherently biased. Everyone has a unique combination of experiences that give them a particular perspective, or bias, and this isn’t necessarily a bad thing. The problems arise when a particular perspective is presented as universal or neutral. If we identify which perspectives are present in our research and, to the best of our ability, which perspectives are absent, we can help people who visit our work realize how they should adapt it to suit their context. Adopting the bias-aware methodology requires a mindset shift, where the human element has just as much weight as the technological element. We must set project timelines and funding models that allow for collaboration with adequately diverse groups of people.
For more on why and how to use a bias-aware NLP research methodology, check out the published paper in the ACL Anthology or read the preprint on ArXiv!
Citation:
Havens, Lucy, Melissa Terras, Benjamin Bach, and Beatrice Alex. 2020 “Situated Data, Situated Systems: A Methodology to Engage with Power Relations in Natural Language Processing Research.” Proceedings of the Second Workshop on Gender Bias in Natural Language Processing. Barcelona, Spain (Online), December 13, 2020, pp. 107-124. Association for Computational Linguistics. Available: https://www.aclweb.org/anthology/2020.gebnlp-1.10
By Lucy Havens
EdIE, a suite of Information Extraction tools for stroke related phenotypes
We are delighted to announce that our tools for Information Extraction from radiology reports are now available on GitHub and as a web demo online! Our language processing tools work on clinical text from brain radiology reports to extract information on stroke-related diseases. See a brief introduction to our online demo here.
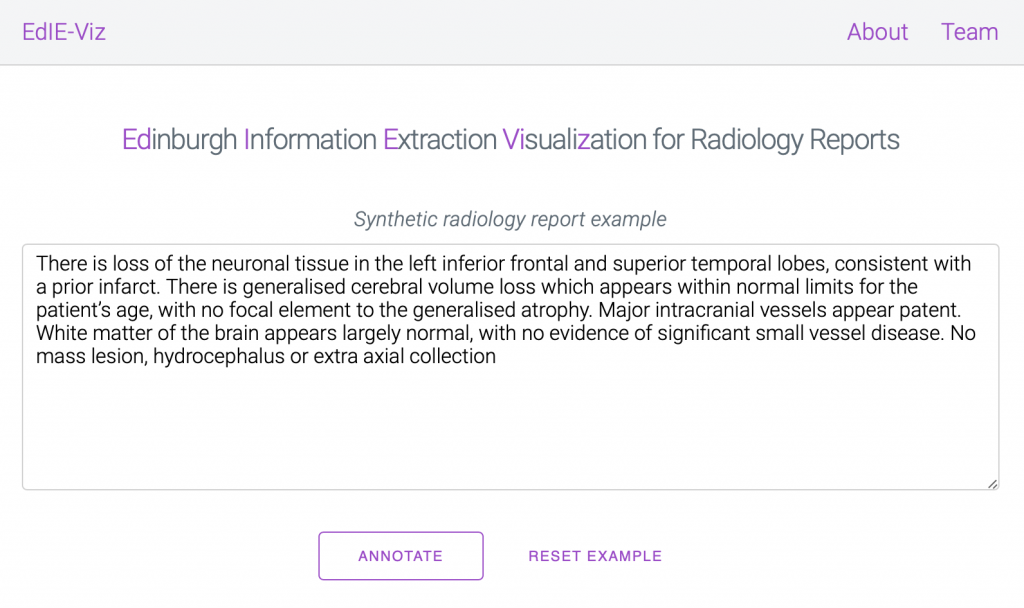
We release these tools in the hope that they will help speed up and improve clinical NLP research in this area by bringing the tools to the data, as in our experience assuming any form of patient data mobility is unrealistic.
We are very happy to collaborate with other researchers on clinical NLP projects, please get in touch for more information. Moreover, if you have any feedback or comments on our tools we would also be very happy to hear from you.
Our LOUHI 2020 workshop paper
The aforementioned tools accompany our workshop paper Not a cute stroke: Analysis of Rule- and Neural Network-based Information Extraction Systems for Brain Radiology Reports (Grivas et al., 2020) that we presented at LOUHI 2020. The recorded presentations will be available soon.
In the paper we discuss our insights from applying information extraction to brain radiology reports with the following three conclusions:
- The choice of metric matters and depends on your downstream task as caveated previously in this blog post by Chris Manning. Directly optimising models for F1 score (the harmonic mean of precision and recall) when doing named entity recognition (NER) has unintended consequences, such as preferring models that predict less entities. This can be seen when breaking down the F1 score into fine-grained error types.
- When relying on semi-automatic annotations, neural language models trained on large corpora can be used to check for any annotation inconsistencies and to get more insights on your annotated data.
- Applying negation detection learned by neural models to new external out-of-sample datasets leads to degrading performance and is still challenging, even in the large pre-trained language model era.
Questions from the audience
What is the reason for the rule-based system performing so well?
There is a lot of generalisable linguistic knowledge as well as medical expert knowledge incorporated in the rules that were developed by Claire Grover after discussions with medical experts. In addition, some mentions are generally straightforward to detect due to the specific vocabulary used. Moreover, the semi-automatic nature of the annotations also further contributes to the increase in scores.
Would you have any thoughts on metrics used for NER?
In the past, ACE and MUC have been used as more fuzzy metrics than the strict metric of the CONLL scorer, but the answer really depends on what our goal is. For hierarchical and fine-grained entity recognition, other metrics that take the hierarchy into account are likely more suitable, for example. Overall, we highlighted that it is beneficial and insightful to break down errors into semantically meaningful groups, especially when comparing models. David S. Batista has a great introduction and discussion of metrics used for NER.
Other papers and talks @LOUHI2020
LOUHI had a lot of interesting related talks, a selection of which we briefly summarise below:
- Hercules Dalianis presented his group’s work on de-identification strategies and their effect on downstream namee published in The Impact of De-identification on Downstream Named Entity Recognition in Clinical (Berg et al., 2020). This is important as decisions taken at the anonymisation stage of clinical text (which is not usually done by researchers) may lead to unforeseen effects at the NLP stage.
- Maciej Wiatrak presented a comparison of multi-task neural network architectures for entity linking published in Simple Hierarchical Multi-Task Neural End-To-End Entity Linking for Biomedical Text (Wiatrak and Iso-Sipila, 2020)
- Aditya Khandelwal‘s presentation was on negation and speculation cue and scope detection as multi-task learning published in Multitask Learning of Negation and Speculation using Transformers (Khandelwal and Britto, 2020).
- Florian Borchert presented work on their corpus of German clinical guidelines which includes structural metadata published in GGPONC: A Corpus of German Medical Text with Rich Metadata Based on Clinical Practice Guidelines (Borcher et al., 2020). This work is of interest to us since we work with clinical guidelines in English for a Clinical Guidelines Browser application.
- Minghao Zhu presented experiments classifying social media posts reflecting the personal experience of the poster published in Identifying Personal Experience Tweets of Medication Effects Using Pre-trained RoBERTa Language Model and Its Updating (Zhu et al., 2020)
- Guergana Savova, the keynote speaker, presented interesting summary of work in this field titled Clinical NLP, some tasks and applications in medicine and many points raised by her rang true to us.
Group thanks and funding
We thank our wider collaborators in the Edinburgh Clinical NLP Group for their help and feedback on this work. We are also very grateful for our funding that made this work possible: the MRC Mental Health Data Pathfinder Award (MRC – MCPC17209) the Alan Turing Institute fellowships and project (EPSRC grant EP/N510129/1), the MRC Clinician Scientist Award (G0902303) and the Scottish Senior Clinical Fellowship (CAF/17/01).
Andreas Grivas, Edinburgh, 18/12/2020
Text Mining Radiology Reports: working group meeting in Edinburgh
Andreas Grivas and Beatrice Alex
At the beginning of March this year, we held a Healtex working group meeting for Text Mining Radiology Reports in the Bayes Centre in Edinburgh. This was an excellent opportunity to bring together teams with a shared focus on improving health services through a better understanding of radiology reports.
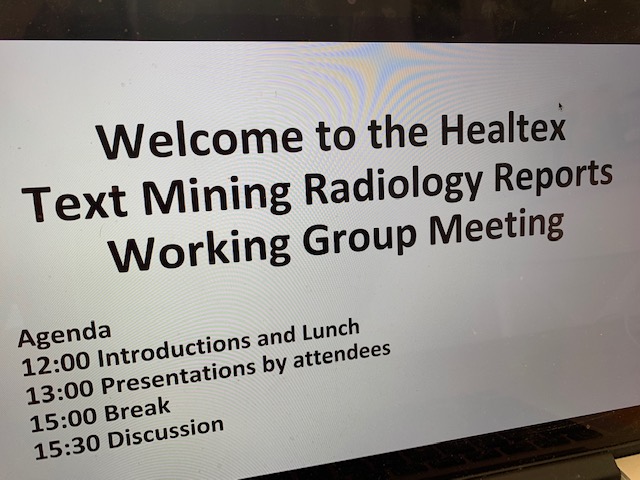
All teams brought different insights and thoughts to the table about challenges they face. We discovered that we have a lot of common ground but also differences in the way we process reports or deal with practical issues. The meeting started with several presentations from attendees which gave us a focus for our discussions.
Dr Beatrice Alex, who chaired the meeting, presented ongoing work at the Edinburgh Language Technology Group on text mining brain imaging reports for stroke type and other observations. She presented the EdIE-R system and a comparison of other machine learning methods for the initial step of recognising different types of named entities in brain imaging reports that are part of the Edinburgh Stroke Study (Jackson et al., 2008) and NHS Tayside data. Dr William Whiteley, consultant neurologist, then presented the use case for this work of conducting large-scale epidemiological research using linked data of electronic healthcare records, e.g. Scotland and Generation Scotland wide. Dr Grant Mair, radiologist by profession, provided a lot of useful practical insights into the process of writing radiology reports, summarising observations and the state of technology used in practice (e.g. speech transcription and checking). Dr Honghan Wu, HDR UK Fellow, then presented an overview of SemEHR, a transfer learning system, which he adapted to the same data as processed by EdIE-R.
We also heard from practitioners using text mining and natural language processing for radiology reports and other types of electronic healthcare records. Dr Peter Hall and Dr. Paul Mitchell from the Cancer Research UK Edinburgh Centre talked about their plans to use text mining for processing pathology reports and explained that one of their challenges is anonymisation to avoid accidental disclosure. Dr Adrian Parry-Jones, Honorary Consultant Neurologist at Salford Royal NHS Foundation Trust, introduced the group to a care bundle for clinicians via an app to reduce mortality of stroke patients. He also proposed ways in which text mining could help direct clinical care. We also heard from Dr Ewen Harrison‘s about the work his group is involved in. They applied a rule-based and a deep learning method to identify mentions of gallstones in MRI scan reports. They found the task to be tractable and achieved high scores against a manually code valuation set. Prof Goran Nenadic, Director of the Healtex network, also informed the group about another Healtex working group focussed on data governance which provides guidance on governance and data sharing related issues.
Some broad topics we discussed were:
Standardising reports and their annotation
- Work on making NHS formats for radiology reports consistent (possible applications for NLP/ML)
- Link annotations to ontologies, e.g. UMLS or SNOMED CT (possibly extend SNOWMED CT UK)
- Crowdsourcing labels (ethics application, inter-annotator agreement)
Experiment results
- Comparing rule-based, machine learning and deep learning methods
- Choosing sample size for statistical power
- Metrics: positive predictive value (precision) vs sensitivity (recall) depends on end goal
Data governance
- Data access
- Best practices in creating and sharing data
- Working with systems on locked down infrastructure
Data privacy and ethics
- Best practices for data anonymisation
- Models not replacing human judgement
Tools and knowledge resources
- UMLS
- SNOMED CT
- GATE
- EdIE-R
- SemEHR
- Other text mining tools
As an outcome of our meeting we discussed ways of working together to avoid duplicating our efforts. We agreed to share our code, systems and rules where possible. We also decided to create a mailing list to keep in touch more easily. We also discussed the possibility of a state-of-the-art review on text mining radiology reports. The last comprehensive systematic review of NLP methods and tools supporting practical clinical applications in radiology is that of Pons et al. (2016).
Future goals in terms of text mining technology include extending previous models to work for additional target types and types of scans (transfer learning) as well as exploring summarisation (e.g. see Zhang et al., 2018 on summarising findings of radiology reports) and medical language simplification. Members of our working group will attend the HealTAC 2019 conference on 24/25thof April in Cardiff where we will present the goals of this group. We are also widening participation to other groups or individuals in the UK and world-wide.
Acknowledgements
We thank Healtex for funding this event.
Organiser
Dr. Beatrice Alex, Chancellor’s Fellow and Turing Fellow at University of Edinburgh, leading the Edinburgh Language Technology Group
Attendees
- Dr William Whiteley, Senior Clinical Fellow and Consultant Neurologist, Centre for Clinical Brain Sciences, University of Edinburgh
- Dr Grant Mair, Senior Clinical Lecturer in Neuroradiology and Radiologist, Centre for Clinical Brain Sciences, University of Edinburgh
- Prof Goran Nenadic, Manchester Institute for Biotechnology, School of Computer Science, University of Manchester, Director of the HealTex Network
- Dr Adrian Parry-Jones, NIHR Clinician Scientist at the University of Manchester and an Honorary Consultant Neurologist at Salford Royal NHS Foundation Trust
- Dr Ewen Harrison, Senior Lecturer, General Surgery, University of Edinburgh and Consultant HPB / Transplant Surgeon, Royal Infirmary of Edinburgh
- Cameron Fairfield, Edinburgh Surgery Online Clinical Research Fellow and PhD Student, Clinical Surgery, Royal Infirmary of Edinburgh
- Dr Riinu Ots, Senior Data Manager, Surgical Informatics, Usher Institute
- Dr Honghan Wu, HDR UK Fellow, Usher Institute, University of Edinburgh
- Andreas Grivas, Research Assistant, Edinburgh Language Technology Group, Institute for Language, Cognition and Computation, School of Informatics, University of Edinburgh
- Richard Tobin, Research Fellow, Edinburgh Language Technology Group, Institute for Language, Cognition and Computation, School of Informatics, University of Edinburgh
- Paul Mitchell, IT Developer, Cancer Research UK Edinburgh Centre, University of Edinburgh
- Dr Peter Hall, Senior Clinical Lecturer, Cancer Research UK Edinburgh Centre, University of Edinburgh
References
Jackson, C., Crossland, L., Dennis, M., Wardlaw and J., Sudlow, C. (2008). Assessing the impact of the requirement for explicit consent in a hospital-based stroke study. QJM: Monthly Journal of the Association of Physicians, 101(4), 281–289.
Pons, E., Braun, L.M., Hunink, M.M. and Kors, J.A. (2016). Natural language processing in radiology: a systematic review, Radiology, 279, pp. 329-343, https://pubs.rsna.org/doi/10.1148/radiol.16142770.
Zhang, Y., Ding, D.Y., Qian, T., Manning, C.D. and Langlotz, C.P.: Learning to summarize radiology findings (2018). In: Proceedings of the Ninth International Workshop on Health Text Mining and Information Analysis, pp. 204-213. Association for Computational Linguistics, Brussels, Belgium (2018), http://aclweb.org/anthology/W18-5623